Rapid Genomic Surveillance of Streptococcus pyogenes Using a No-Code Platform
Streptococcus pyogenes, also known as group A Streptococcus, causes infections ranging from mild throat infections to life-threatening and devastating conditions like necrotizing fasciitis and septic shock. It is most common in children and adolescents, and causes particularly high disease burden in low- and middle-income countries, causing particularly high rates of illness and death in resource-limited regions.
Seeking to better understand the genomic epidemiology of this dangerous pathogen, a research team led by Sébastien Boutin obtained 76 genomes from Gabon and analyzed them. The results were published recently in ASM Microbiology Spectrum.
In this post, we will recreate parts of the genomic epidemiology investigation using Solu platform, a no-code tool, in just minutes.
What we did
We obtained the 76 genomes from this dataset from NCBI and uploaded them to the Solu platform. Solu automatically initiated analyses and characterized the samples.
Visit https://platform.solugenomics.com/w/solu-s-pyogenes-1/ to see the live workspace!
Results
Genomic characterization
Most analyses were completed successfully. However, the MLST assignment couldn't be made for several samples. The Solu platform automatically flagged these samples' original assemblies as having more than 200 contigs. MLST failure can stem from limitations in the scheme, tool parameters, or the quality of the original sequencing and assembly.

The Solu platform found the resistance genes tet(L), tet(M), and thfT from this dataset. 64 isolates exhibited the tet(M) gene, which suggests tetracycline resistance is prevalent in this region.
.png)
Phylogenetics
Solu automatically generated a phylogenetic tree and identified 16 clonal clusters, suggesting potential transmission events.
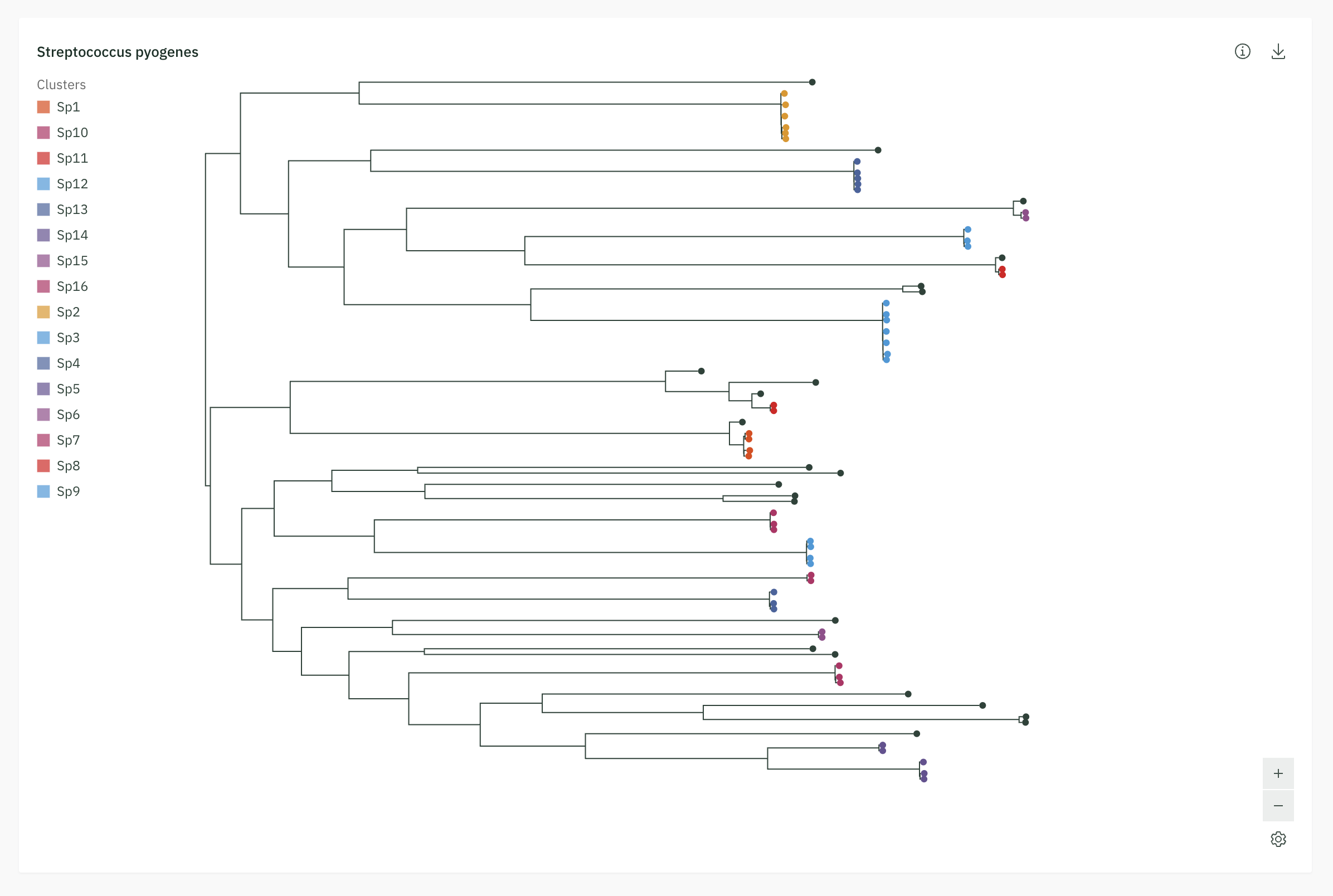
This out-of-the-box analysis closely matched the findings of the 2024 article, which employed a similar SNP-based method for sample clustering.

Agreement with the 2024 publication
How does Solu's output compare to the original publication? Let's examine the results.
For MLST, we saw 87% agreement. Solu classified 9 isolates as ST1186, which the original paper didn't assign to any type. The original article classified sample G0673S as ST565, while the Solu platform couldn't classify it due to a missing mutS allele in the genome. The ST1186 difference might be explained by variations in methodology and scheme versions, and the mutS issue could potentially be resolved by creating a higher-quality assembly.
Antibiotic Resistance Genes had near identical results, agreement was 100% for tet(L), 97% for tet(M) and 100% for thfT.
The lmrP gene wasn't included in our results as it's not present in the AMR database we used.
Both analyses found 16 potential transmission clusters. The cluster assignment agreement was 93%. The small difference came from Solu using a less strict threshold (20 vs 18), which placed two samples in a cluster that the original study had labeled as singletons.
Conclusion
By using Solu platform for automated microbial genome analysis, we were able to rapidly:
- Identify 16 distinct clonal clusters within the sample set
- Flag potential quality issues for some samples
- See the prevalence of tetracycline resistance genes
- Generate a phylogenetic tree
All results with very high agreement with a peer-reviewed publication prepared by an expert team.
We hope that this quick post can show how tools like Solu platform can democratize complex genomic analyses, making them accessible to researchers without extensive bioinformatics expertise. Access to data can have significant public health implications, enabling rapid response to outbreaks and more informed decision-making.
Get started for free
Create your free Solu Platform account today to start analyzing genomes.